
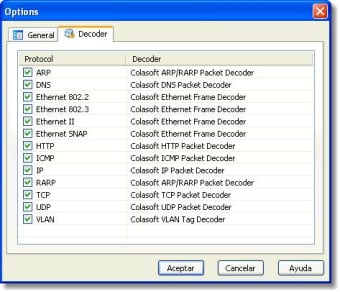
15.6.4 illustrates how this method facilitates the extraction of bearing-fault-related components from a signal while rejecting the unwanted harmonics.
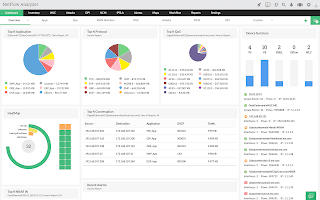
The network is then trained using wavelet packets characterizing the above-mentioned types of faults. These parameters are kurtosis (a measure of spikiness) and the spectrum peak ratio (an indication of the presence of localized defects). Before the neuro-fuzzy network is trained (using wavelet packets extracted from vibration signals), suitable input parameters to train the network are selected. ANFIS is a transformational model of integration where the final fuzzy inference system is optimized via artificial neural network training. Hence a continuous function can now be represented as a linear combination of orthogonal wavelet packet functions as follows:įeature Extraction: The extraction of high-frequency transients due to bearing impact resonance is achieved via best-basis DWPA representation using the Daubechies wavelet of order 20 and an adaptive network-based fuzzy inference system (ANFIS). We still have the dilation (j) and translation (k) indices, but there is also an oscillation index (b). Now that we speak of wavelet packet functions we need to add another index. We will let W(t) represent a wavelet packet function. In addition to these functions, we also have wavelet packet functions, so a function f(t) can now be represented as a sum of orthogonal wavelet packet functions. We still have the scaling function ϕ(t), and the wavelets generated from the mother wavelet ψ(t). We recall from wavelet analysis that the scaling function ϕ(t) plays a key role, in that the wavelet ψ(t) is generated from the scaling function. Because wavelet packet analysis filters high frequency components as well as the low frequency components, wavelet packets are more suited to representing functions that have oscillatory or periodic behaviour.
